Artificial intelligence in the curricula of postgraduate studies in financial management: Survey results
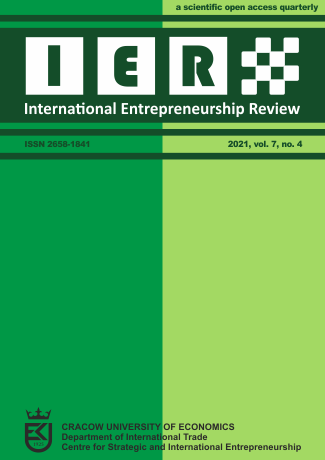
Abstract
Objective: The aim of this article is to determine the awareness, preferences, significance, and effectiveness of application of artificial intelligence (AI) among participants of postgraduate studies in the field of financial management.
Research Design & Methods: The pilot study was conducted with the use of a survey, and the findings were analysed using the Importance-Performance Analysis (IPA) method. The survey group was composed of individuals working on final projects developed within the framework of postgraduate studies in finance management, carried out in collaboration with e.g. ACCA, CIMA, CFA.
Findings: The obtained findings have identified a demand for the incorporation of knowledge in the field of AI in the process of education in the area of finance management. The survey results will be used to modify the content of the curricula adopted for postgraduate studies in the field of finance management.
Implications & Recommendations: The only chance for experienced professionals and managers to gain knowledge about AI is to enrol in postgraduate studies. The existing postgraduate curricula are flexibly modified, which makes it possible to incorporate the knowledge about state-of-the-art IT solutions and AI on an ongoing basis. This requires, however, permanent research into the needs of the developing market.
Contribution & Value Added: The progressing digitalisation in the socio-economic sphere of our life translates into huge amounts of data being transmitted, collected, and stored, and calls for a need to implement new technologies and solutions utilising techniques and algorithms based on artificial intelligence (AI). The SARS-CoV-2 pandemic has increased the demand for modern IT solutions in the field of e.g. finance management.
Keywords
artificial intelligence (AI), education in financial management, curriculum in postgraduate education
Author Biography
Mariusz Andrzejewski
Associate Professor, Head of the Department of Financial Accounting, Institute of Finance, Cracow University of Economics (Poland). Habilitated doctor in economics (2013). PhD in economics (2001). Master of Science in informatics (1997). Master in accounting (1996). His research interests include financial audit, corporate finance, financial and managerial accounting, computer-aided techniques in accounting.
Patryk Dunal
Assistant Professor at the Department of Financial Accounting, Institute of Finance, Cracow University of Economics (Poland). PhD in economics (2018). Master in finance and accounting (2014). Bachelor in finance and accounting (2012). His research interests include corporate finance, fuel and energy market, managerial accounting, financial statements analysis, accounting of derivatives, risk management.
References
- Biletska, O., Biletskiy, Y., Li, H., & Vovk, R. (2010). A semantic approach to expert system for e-assessment of credentials and competencies. Expert Systems with Applications, 37(10), 7003-7014.
- Dai, W.Z., Xu, Q., Yu, Y., & Zhou, Z.H. (2019). Bridging Machine Learning and Logical Reasoning by Abductive Learning. Proceedings of 33rd Conference on Neural Information Processing System, 2-5.
- Dawes, P.L., & Patterson, P.G. (1987). An Empirical Study of Product Management in Australia. Australian Journal of Management, 12 (2), 237-262.
- DeCarlo, P., & Rizk, N. (2010). The design and development of an expert system prototype for enhancing exam quality. International Journal of Advanced Corporate Learning, 3(3), 10-13.
- Grabiński, K., & Wójtowicz, P. (2019). Earnings quality, earnings management and religiosity: A literature review. International Entrepreneurship Review, 5(4), 41-57. https://doi.org/10.15678/IER.2019.0504.03
- Graf, L.A., Hemmasi M., & Nielsen, W. (1992). Importance-Satisfaction Analysis: A Diagnostic Tool for Organizational Change. Leadership & Organization Development Journal, 13(6), 8-12. https://doi.org/10.1108/01437739210021857
- Jiang, Y., Bosch, N., Baker, R. S., Paquette, L., Ocumpaugh, J., Andres, J. Ma. A. L., Moore, A. L., & Biswas G. (2018). Expert feature-engineering vs. deep neural networks: which is better forsensor-free affect detection? In: Penstein Rosé, C., Martínez-Maldonado, R., Hoppe, H. U., Luckin, R., Mavrikis,M., Porayska-Pomsta, K., McLaren, B., du Boulay B. (eds.) Artificial Intelligence in Education. AIED 2018. Lecture Notes in Computer Science. Vol. 10947, pp.198-211. Cham: Springer.
- Kardan, A.A., Sadeghi, H., Ghidary, S.S., & Sani, M.R.F. (2013). Prediction of student course selection in online higher education institutes using neural network. Computers and Education, 65, 1-11.
- Klement, E.P., & Slany, W. (1993). Fuzzy Logic in Artificial Intelligence. In: Proceedings of the 8th Austrian Artificial Intelligence Conference, LNAI 695, Springer.
- Kose, U., & Arslan, A. (2016). Intelligent e-Learning system for improving students’ academic achievements in computer programming courses. International Journal of Engineering Education, 32(1, A), 185-198.
- Martilla, J.A., & James, J.C. (1977). Importance-Performance Analysis. Journal of Marketing, 41(1), 77-79.
- Matayoshi, J., Uzun, H., & Cosyn, E.: Deep (2019). (Un)Learning: Using Neural Networks to Model Retention and Forgetting in and Adaptive Learning System. In: Isotani S., Millán E., Ogan A., Hastings P., McLaren B., Luckin R. (eds.), Artificial Intelligence in Education. AIED 2019. Lecture Notes in Computer Science. Vol 11625, pp. 258-260. Cham: Springer.
- Rau, M.A., Sen, A., & Zhu, X. (2019). Using Machine Learning to Overcome the Expert Blind Spot for Perceptual Fluency Trainings. In: Isotani S., Millán E., Ogan A., Hastings P., McLaren B., Luckin R. (eds.), Artificial Intelligence in Education. AIED 2019. Lecture Notes in Computer Science. Vol. 11625, pp. 406-408. Cham: Springer.
- Sanchez, E. L., Santos-Olmo, A., Alvarez, E., Huerta, M., Camacho, S., & Fernandez-Medina, E. (2016). Development of an expert system for the evaluation of students’ curricula on the basis of competencies. Future Internet, 8(2), 22. https://doi.org/10.3390/fi8020022
- Sieja, M., & Wach, K. (2019). The Use of Evolutionary Algorithms for Optimization in the Modern Entrepreneurial Economy: Interdisciplinary Perspective. Entrepreneurial Business and Economics Review, 7(4), 117-130. https://doi.org/10.15678/EBER.2019.070407
- Sun, K.T., Chen, Y.J., Tsai, S.Y., & Cheng, C.F. (2008). Creating IRT-based parallel test forms using the genetic algorithm method. Applied Measurement in Education, 21(2), 141-161. https://doi.org/10.1080/08957340801926151
- Zhou, Z.H. (2019). Adductive learning: towards bridging machine learning and logical reasoning. Science China Information Sciences, 62(7), 076101. https://doi.org/10.1007/s11432-018-9801-4