Mutual information between the main foreign subindices: The application of copula entropy around WHO’s declaration date at the time of the COVID-19 pandemic
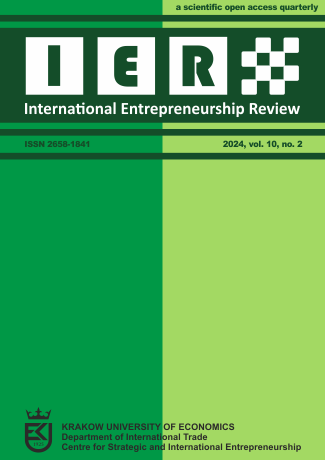
Abstract
Objective: The objective of this article is to investigate the dependencies between selected European subindices before and during the COVID-19 pandemic.
Research Design & Methods: The main analysis was quantitative. We used copula entropy and Pearson’s correlation. We considered the closing prices of sectoral indices from France (CAC sectors), Germany (DAX sectors), the UK (FTSE sectors), and the US (SP sectors), along with the main indices from these countries, that is CAC40, DAX, SP500, and FTSE100 (we collected the data from the database investing.com for the period from 4 January 2017 to 30 March 2023). We performed all analyses using R along with supplementary packages.
Findings: When it comes to indications of the strength of dependence before and after the event (the outbreak of the COVID-19 pandemic) in relation to mutual information (delta) and linear correlation, we saw the biggest differences for the German market. For the DAX sectors, linear correlation underestimates post-event dependencies. The dependencies for other countries were similar on average. For half of the sectors (all markets), we recorded an increase in dependence after the event. A sector where we recorded growth in all countries was the TECH sector.
Implications & Recommendations: The dependence measurement using mutual information expressed in terms of copulas has many advantages. It is not limited to measuring linear correlations. It can also capture a nonlinear correlation. Furthermore, it not only measures the dependence degree, but also considers the dependence structure, which is more than a correlation. Moreover, there was no assumption about the ellipticity of marginal and joint distribution. This dependence measure even allows for the modelling of the dependence of variables with different cumulative distribution functions.
Contribution & Value Added: The novelty of this article is that it compares the results of dependence measurements by linear correlations and mutual information expressed in terms of copula entropy. Considering the indices and subindices of the main European stock markets, when both measures of dependence were used, we obtained significantly different results in both subperiods under investigation (i.e. before and after March 11, 2020).
Keywords
foreign main subindices, pandemic Covid-19, mutual information, copula entropy, linear correlation
Author Biography
Henryk Gurgul
Professor at AGH University of Science and Technology in Krakow, Department of Applications of Mathematics in Economics. His research interests include econometrics, financial econometrics, international financial markets, and input-output models. Author of more than 200 publications. Visiting professor at universities in Graz and Klagenfurt (Austria), Erlangen-Nuernberg, Greifswald, Ilmenau, Saarbruecken (Germany), Trieste and Siena (Italy), Valencia (Spain), Koper (Slovenia), Joensuu (Finland). Honours: Awarded in 2007 the most prestigious prize for economics in Poland Bank Handlowy w Warszawie S.A. Award (Citi Bank), in 2015 awarded the Medal of Honour, University of Graz, Austria.
Robert Syrek
PhD, Assistant Professor at the Institute of Economics, Finance and Management, Faculty of Management and Social Communication at the Jagiellonian University in Krakow. His main areas of research interest include time series analysis and forecasting, financial econometrics and modelling the dependence structures of financial time series (especially using copula functions).
References
- Albulescu, C.T. (2020). COVID-19 and the United States financial markets’ volatility. Finance Research Letters, 38. https://doi.org/10.1016/j.frl.2020.101699
- Alomari, M., Power, D.M., & Tantisantiwong, N. (2018). Determinants of equity return correlations: a case study of the Amman Stock Exchange. Review of Quantitative Finance and Accounting, 50(1), 33-66. https://doi.org/10.1007/s11156-017-0622-4
- Ashraf, B.N. (2020). Stock markets’ reaction to COVID-19: cases or fatalities?. Research in International Business and Finance, 54. https://doi.org/10.1016/j.ribaf.2020.101249
- Aslam, F., Awan, T.M., Syed, J.H., Kashif, A., & Parveen, M. (2020a). Sentiments and emotions evoked by news headlines of coronavirus disease (COVID-19) outbreak. Humanities and Social Sciences Communications, 7, 23. https://doi.org/ 10.1057/s41599-020-0523-3
- Aslam, F., Mohti, W., & Ferreira, P. (2020b). Evidence of intraday multifractality in European stock markets during the recent coronavirus (COVID-19) outbreak. International Journal of Financial Studies, 8(2), 31. https://doi.org/0.3390/ijfs8020031
- Aslam, F., Aziz, S., Nguyen, D.K., Mughal, K.S., & Khan, M. (2020c). On the efficiency of foreign exchange markets in times of the COVID-19 pandemic. Technological Forecasting and Social Change, 161. https://doi.org/10.1016/j.techfore.2020.120261
- Bakas, D., & Triantafyllou, A. (2020). Commodity price volatility and the economic uncertainty of pandemics. Economics Letters, 193. https://doi.org/10.1016/j.econlet.2020.109283
- Barberis, N., Shleifer, A., & Wurgler, J. (2005). Comovement. Journal of Financial Economics, 75(2), 283-317. https://doi.org/10.1016/j.jfineco.2004.04.003
- Chiang, T.C., & Zheng, D. (2010). An empirical analysis of herd behaviour in global stock markets. Journal of Banking & Finance, 34(8), 1911-1921. https://doi.org/10.1016/j.jbankfin.2009.12.014
- Goodell, W. (2020). COVID-19 and finance: agendas for future research. Finance Research Letters, 35. https://doi.org/10.1016/j.frl.2020.101512
- Ji, J., Huang, Ji, C., Cao, Y., & Hu, S. (2019). The network structure of Chinese finance market through the method of complex network and random matrix theory. Concurrency and Computation: Practice and Experience, 31(9). https://doi.org/10.1002/cpe.4877
- Joe, H. (1989) Relative entropy measures of multivariate dependence. Journal of the American Statistical Association, 84(405), 157-164. https://doi.org/10.1080/01621459.1989.10478751
- Kodres, E., & Pritsker, M. (2002). A rational expectations model of financial contagion. The Journal of Finance, 57(2), 769-799. https://doi.org/10.1111/1540-6261.00441
- Leduc, S., & Liu, Z. (2020). The uncertainty channel of the coronavirus. FRBSF Economic Letter, 7, 1-5. Retrieved from https://www.frbsf.org/economic-research/files/el2020-07.pdf on October 2, 2023.
- Long, W., Guan, L., Shen, J., Song, L., & Cui, L. (2017a). A complex network for studying the transmission mechanisms in stock market. Physica A: Statistical Mechanics and Its Applications, 484, 345-357. https://doi.org/10.1016/j.physa.2017.04.043
- Long, W., Tang, Y., & Cao, D. (2016). Correlation analysis of industry sectors in China’s stock markets based on interval data. Filomat, 30(15), 3999-4013. https://doi.org/10.2298/FIL1615999L
- Long, H., Zhang, J., & Tang, N. (2017b). Does network topology influence systemic risk contribution? A perspective from the industry indices in Chinese stock market. PLoS One, 12(7). https://doi.org/10.1371/journal.pone.0180382
- Mazur, M.D., & Vega, M. (2020). COVID-19 and the March 2020 stock market crash. evidence from S&P1500. Finance Research Letters, 38. https://doi.org/10.1016/j.frl.2020.101690
- Ma, J., & Sun, Z. (2011) Mutual Information Is Copula Entropy. Tsinghua Science & Technology, 16(1), 51-54.
- Nelsen, R.B. (2006). An Introduction to Copulas. New York: Springer.
- Okorie, D.I., & Lin, B.Q. (2020). Stock markets and the COVID-19 fractal contagion effects. Finance Research Letters, 38. https://doi.org/10.1016/j.frl.2020.101640
- Qiao, H., Xia, Y., & Li, Y. (2016). Can network linkage effects determine return? Evidence from Chinese stock market. PLoS One, 11(6), https://doi.org/10.1371/journal.pone.0156784
- Poynter, J.G., Winder, J.P., & Tai, T. (2015). An analysis of comovements in industrial sector indices over the last 30 years. Review of Quantitative Finance and Accounting, 44(1), 69-88. https://doi.org/10.1007/s11156-013-0399-z
- Rizwan, S., Ahmad, G., & Ashraf, D. (2020). Systemic risk: the impact of COVID-19. Finance Research Letters, 36. https://doi.org/10.1016/j.frl.2020.101682
- Sharif, A., Aloui, C., & Yarovaya, L. (2020). COVID-19 pandemic, oil prices, stock market, geopolitical risk and policy uncertainty nexus in the US economy: fresh evidence from the wavelet-based approach. International Review of Financial Analysis, 70. https://doi.org/10.1016/j.irfa.2020.101496
- Shehzad, K., Xiaoxing, L., & Kazouz, H. (2020). COVID-19’s disasters are perilous than global financial crisis: a rumor or fact?. Finance Research Letters, 36. https://doi.org/10.1016/j.frl.2020.101669
- Sukcharoen, K., & Leatham, D.J. (2016). Dependence and extreme correlation among US industry sectors. Studies in Economics and Finance, 33(1), 26-49. https://doi.org/10.1108/SEF-01-2015-0021
- Surya, C., Natasha, G., & Natasha, G. (2018). Is there any sectoral cointegration in Indonesia equity market?. International Research Journal of Business Studies, 10(3), 159-172. https://doi.org/10.21632/irjbs.10.3.159-172
- Tenzer, Y., & Elidan, G. (2016). On the Monotonicity of Copula Entropy. https://doi.org/10.48550/arXiv.1611.06714
- Wang, X.D., & Hui, X.F. (2018). Cross-sectoral information transfer in the Chinese stock market around its crash in 2015. Entropy, 20(9), 1-14. https://doi.org/10.3390/e20090663
- Zaremba, A., Kizys, R., Aharon, D.Y., & Demir, E. (2020). Infected markets: novel coronavirus, government interventions, and stock return volatility around the Globe. Finance Research Letters, 35. https://doi.org/10.1016/j.frl.2020.101597
- Zhang, D., Hu, M., & Ji, Q. (2020). Financial markets under the global pandemic of COVID-19. Finance Research Letters, 36. https://doi.org/10.1016/j.frl.2020.101528
- Zhao, N., & Lin, W. (2011). A copula entropy approach to correlation measurement at the country level. Applied Mathematics and Computation, 218(2), 628-642. https://doi.org/10.1016/j.amc.2011.05.115